Analyzing learning progressions
How data from digital learning environments can be used to support students’ learning
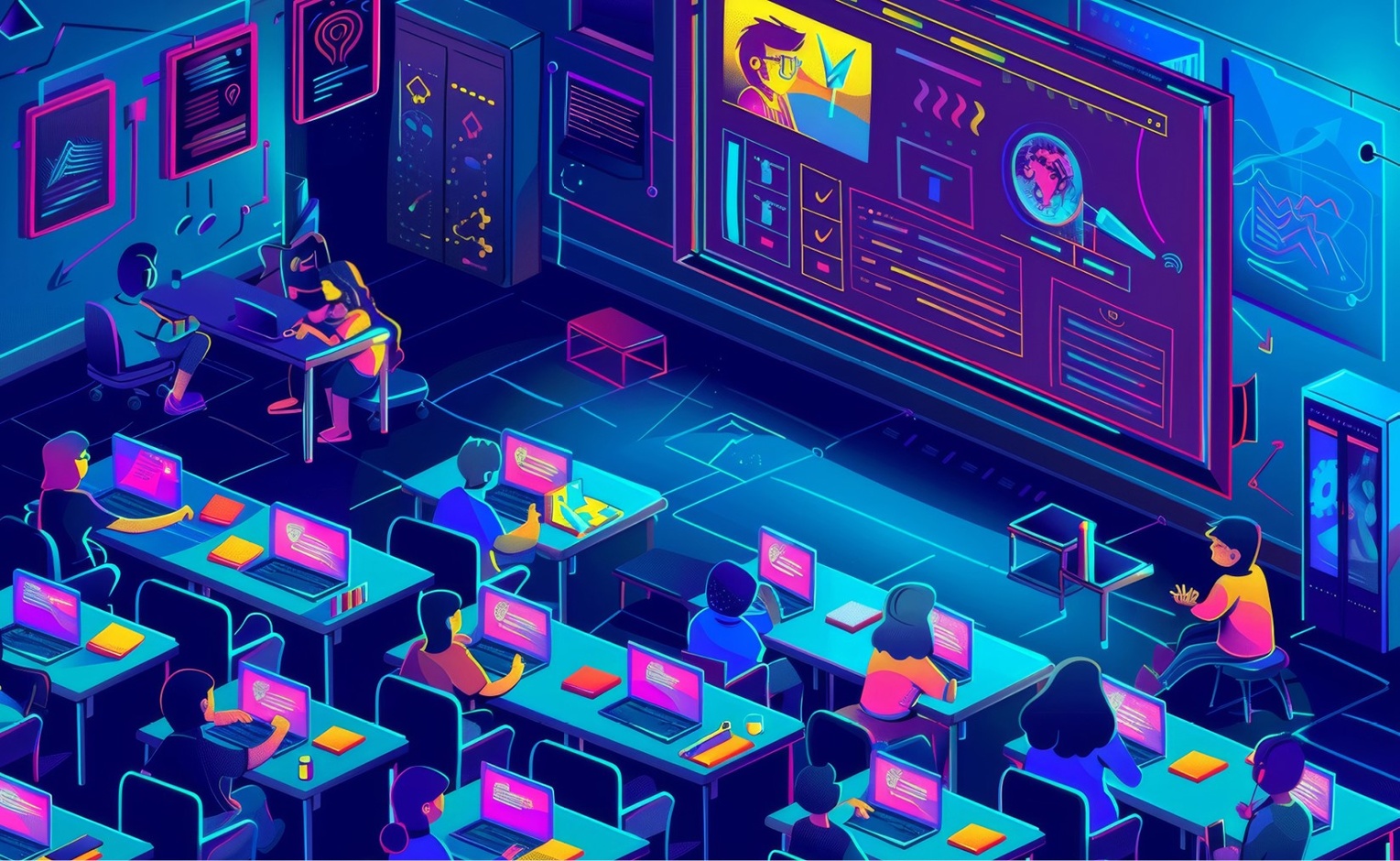
Marcus Kubsch & Knut Neumann
The COVID-19 pandemic has clearly shown how important digital technologies are for learning in schools. This highlights the efforts made in recent years to expand the use of digital technologies in the classroom. It's not simply a matter of providing worksheets via teaching and learning platforms or using digital media such as simulations more frequently. Digital technologies also enable us to rethink some aspects of teaching and learning.
In physics classrooms for example, digital measurement systems allow to capture aspects of phenomena that are hard to capture with traditional analog measurement systems and make it easy to analyze data in a way that actually mirrors how data is actually analyzed in research and industry. However, the real potential of digital technologies lies elsewhere:
Extensive data about students’ learning is generated when students are working with them. This initially includes the products generated by students when working on tasks, such as the measured values recorded. However, data is also recorded as part of the task completion process, e.g. in which order which measured values were recorded. The "Learning Progression Analytics" (LPA) research area at the IPN investigates how the data generated when working with digital technologies can be used to reconstruct students’ learning progressions, determine the effectiveness of learning progressions with regard to the development of targeted skills and knowledge, and competencies and identify causes that inhibit learning with the goal to support students’ learning.
Many believe that digital technologies have great potential to improve teaching and learning. In addition to other benefits, the main expectation is greater individualization and therefore instruction tailored to the needs of individual students. For example, a teacher can assign specific tasks to different groups of students or even individual students in a digitally enhanced learning environment.
Two prerequisites are necessary, however, to use the potential of digital technologies for more individualized instruction:
First, digitally enhanced instructional materials are needed. Digitally enhanced instructional materials are regular materials that use digital technologies to better support students in their learning. For example, this can be done by providing students with a video for each demonstration experiment in their digital workbook for the lesson unit. Students who did not immediately make the correct observation during the demonstration experiment can do so using this method.
Second, the digitally enhanced instructional materials must provide students and teachers with feedback on the learning process - ideally not only on whether individual tasks have been successfully completed, but also in particular on the learning goals targeted in the respective instruction. Research shows that an incorrectly completed task is not necessarily unproductive in terms of learning. On the contrary, it can have a cognitively activating effect and, combined with appropriate instruction, can even lead to improved learning outcomes. However, it is necessary to track the learning trajectory of individual students and evaluate it in terms of its contribution to (long-term) learning outcomes to provide them and teachers with feedback.
The AFLEK project is a joint project with the Leibniz Institute for Research and Information in Education (working group of Prof. Dr. Hendrik Drachsler) and the Ruhr University Bochum (working group of Prof. Dr. Nikol Rummel) to investigate the extent to which learning trajectories can be reconstructed and whether the feedback of information on learning trajectories to teachers actually leads to improved learning outcomes. The AFLEK project builds on the energie.TRANSFER project. In the energie.TRANSFER project a total number of twelve instructional units were developed, the aim of which was to support students in developing a well-organized knowledge of energy. These short units are designed to be seamlessly integrated into existing curricula in schools. Based on an interesting and personally relevant question (e.g. Why does a laptop sometimes gets hot?), the students gradually develop the understanding they need to answer the question. They use scientific practices such as constructing and using models, planning and conducting investigations, analyzing data and engaging in argument from evidence.
The units were designed as digitally enhanced instructional materials and implemented in the Moodle learning management system. Part of the reason for this was to enable teachers to easily adapt the units. The units therefore included additional information on physics teaching and learning, such as the didactic objectives of the individual teaching activities and explanations on the choice of methods and media. This additional information was only available to the teachers. Another advantage of implementing the units digitally, was the ability to individualize lessons. This applies, for example, to videos of experiments previously carried out by the teacher in class and for which the students were asked to answer questions. Another example is the use of digital tools to create models. This allows for the simple representation of complex relationships. The implementation in Moodle allows seamless integration with other tasks, for example, results from a modelling activity can easily be transferred when using the model to develop an argument about what is the best way to mount solar cells on a roof. It also facilitates the automated evaluation of the artifacts generated by the students during the activities - essentially open-ended answers. However, the evaluation of such answers is not trivial, as classic evaluation strategies such as the detection of certain keywords are prone to errors. Spelling errors or paraphrasing can lead to incorrect evaluations. Artificial intelligence methods (such as natural language processing (NLP) and machine learning (ML)) can help to address this issue.
Research into machine learning has made great progress in recent decades. Today, machine learning is used in many everyday applications, including the recognition of spoken language, the operation of digital assistants and online shopping recommendations. Despite further developments, the machine evaluation of free text answers is not without its problems, especially when it comes to evaluating the answers not only as correct or incorrect, but to detect certain conceptual ideas or misconceptions. This was precisely the aim of the AFLEK project. Only when it is clear what knowledge students already have and what knowledge is still lacking can adjustments be made to develop the learning outcomes. Simply assessing whether a task has been completed correctly or incorrectly does not suffice.
NLP methods are generally used when evaluating open text responses by machine. One problem here is the length of the answers. Short answers are more difficult to process. This becomes even more of a problem when not just a single criterion (e.g. correctness), but several criteria such as different specific ideas need to be automatically assessed. This is why so-called transformer models were used in the project. These models are based on neural networks and combine the advantages of improved training speed and consistent prediction quality with greater sensitivity to (linguistic) context.
As part of the AFLEK project, the students' answers to two units from the energie.TRANSFER project were first laboriously coded by trained staff in order to train the machine learning models. The presence of various physics concepts - e.g. the concept of forms of energy (such as electrical energy or thermal energy) or the concept of energy transformations (such as the transformation of electrical energy into thermal energy) - was coded for each answer.
The models trained in this way can now be used to reconstruct the development of the students' knowledge - in terms of the existence of certain subject-related ideas and links between them.
This reconstruction can be imagined as knowledge networks. The development of these knowledge networks was observed in an experiment with two students, referred to below as person A and person B. It was investigated which knowledge elements the persons used in the tasks that had been completed by the time the networks were created. It was found that neither person made any connections between the knowledge elements at the beginning of the teaching unit. In the course of the teaching unit, a connection between the knowledge elements of thermal energy and electrical energy became clear. At this point, the participants were able to use these knowledge elements together in tasks, e.g. by describing that the two forms of energy can be observed in a phenomenon. In addition, there was a clear difference between the two people as they progressed: While person A linked the knowledge on energy conversion to the two forms of energy and successfully applied this knowledge in the tasks completed, this was not the case for person B. Person B only applied the knowledge about the forms of energy, but not about the energy conversion. It was observed that the individuals did not integrate any further ideas into their knowledge networks, but were still able to successfully use and thus consolidate their existing knowledge.
How do these knowledge networks benefit the classroom? They provide both differentiated and intuitively accessible information about the students' knowledge. In the example above, it is clear that student A and B do not make a connection between forms of energy and associated quantities - the so-called indicators for the presence of energy of a certain form - until the end of the unit. Based on this information, the teacher could reiterate that it is important for the explanation of phenomena not only to name which forms of energy are transformed into each other, but also how this can be recognized. From a research perspective, the exciting question arises as to what extent these connections are relevant for the development of scientific competence at all - as is commonly assumed in educational research. The "Learning Progression Analytics" research group is currently investigating these and similar questions.
The ALICE project, in cooperation with the Leibniz Institute for Research and Information in Education (working group of Prof. Dr. Hendrik Drachsler), the Ruhr University Bochum (working group of Prof. Dr. Nikol Rummel) and the Leibniz Institute for Knowledge Media (working group of Prof. Dr. Ulrike Cress), is analyzing learning progressions in significantly longer instructional units of around ten weeks in mathematics and science. This is based on the approach developed in the AFLEK project.
In summary, the Learning Progression Analytics working group uses artificial intelligence methods, especially machine learning, to first reconstruct students’ knowledge and competencies from product data (e.g. student responses) and process data (e.g. video playback behavior) from digital learning environments. Individual learning trajectories are then reconstructed based on this information and evaluated in terms of their success in terms of desired learning outcomes. This will create a basis for providing students with automated and individualized support in their learning in the future. Examples of this include providing tailored feedback to students and teachers or even suggesting additional tasks or instructional strategies. Particularly in times of (increasing) teacher shortages, the projects in the area of Learning Progression Analytics therefore make an important contribution to ensuring and improving the quality of teaching.
About the authors:
Prof. Dr. Marcus Kubsch was a postdoctoral researcher in the Department of Physics Education at the IPN from 2019 to 2023 and is now Professor of Physics Education at Freie Universität Berlin.
Prof. Dr. Knut Neumann is Director of the Department of Physics Education at the IPN.