Artificial intelligence for social justice in learning
Making artificial intelligence work for all students
Adrian Grimm & Anneke Steegh
The use of artificial intelligence (AI) in physics lessons is increasing - and its application is also effective for learning. Effective learning for everyone? A research group at the IPN is investigating the extent to which bias - i.e. systematic distortions - play a role in the use of AI in physics lessons and affect students' science identity.
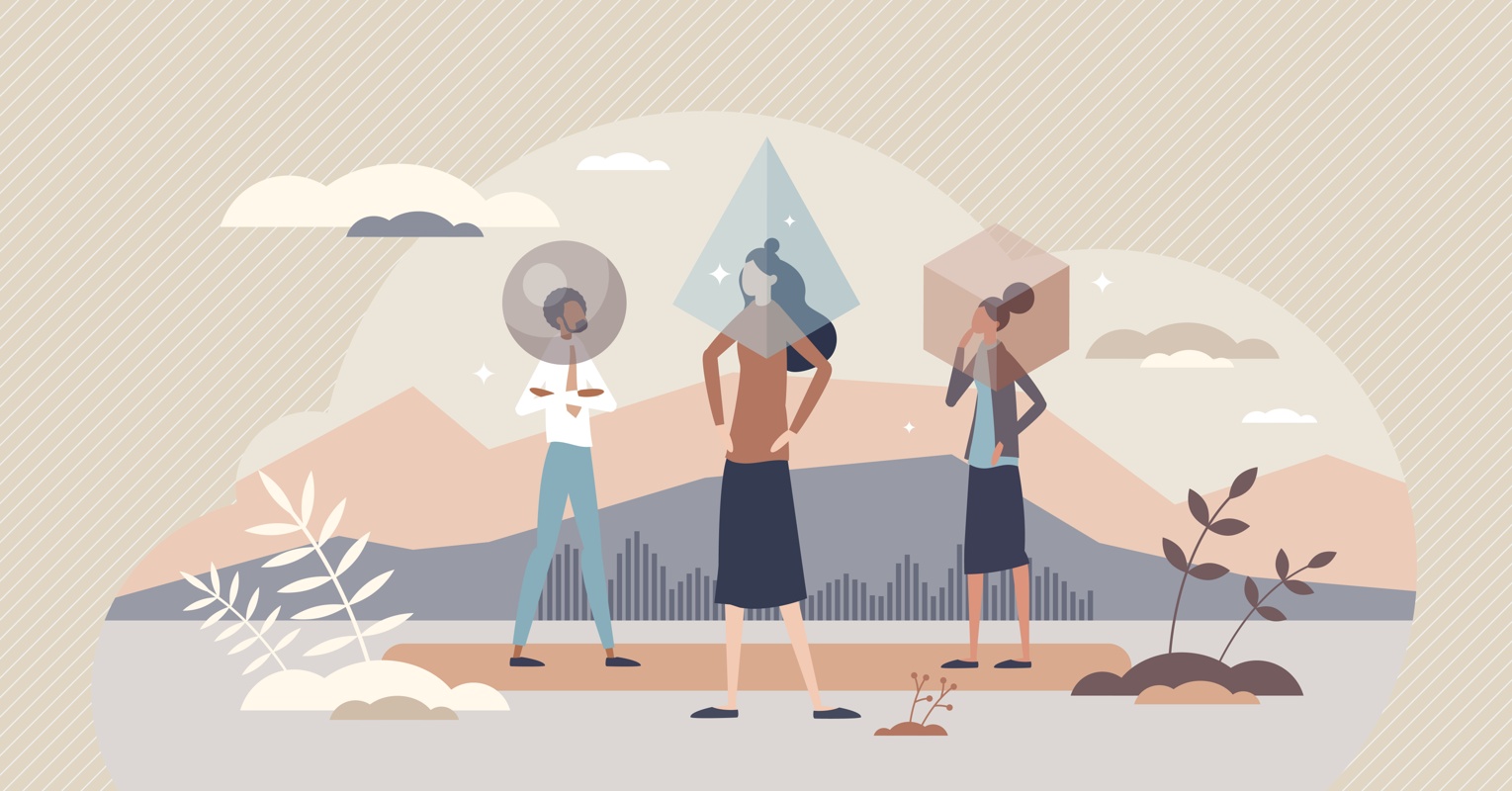
Identity development in the natural sciences, i.e. the positive self-assessment with regard to the statement “I am a scientist”, depends to a large extent on recognition. Recognition from people who are perceived as being professionally competent is especially important here - in the case of students, for example, their physics teachers.
In physics lessons, AI is used, for example, to evaluate students' answers automatically. Often, these evaluations lead directly to feedback for the students or serve as a source of feedback for the teachers, which plays a key role in recognition.
This is why bias is relevant to AI identity
What is bias? Bias in AI is when, for example, AI systematically works better for male students than for female students. This can occur because the AI is trained with historical data. If this data already contains a bias, the AI adopts this bias and ultimately also delivers biased results.
AI working less reliably for certain groups, such as female or non-binary students, can lead to unfounded negative feedback. Affected students then have less opportunity to develop a strong science identity. This in turn can reinforce the inequalities that are already visible today: A large gap is evident along gender identity lines when it comes to professions in which physics plays a central role, such as electrical technician or engineer.
The path to social justice in AI
Andreas Mühling explained how AI training works in his article in IPN Journal No. 11 in a clear and accessible way for beginners. Many codes of conduct that prohibit discrimination exist for practitioners in the field of AI. Numerous research studies also show that bias in AI is not an isolated case, but occurs systematically, and that there is a large gap between the ban on discrimination on paper and what happens in AI practice. A case study was able to show why this gap exists for an example in physics lessons: Specifications on the prohibition of discrimination are often not sufficiently specified. As an AI community, we therefore need to consciously ask ourselves the following questions: What specific biases do we need preventative measures against? More specifically for physics education: Which biases are particularly relevant in physics education and how can we counter them effectively and efficiently with political regulation?
An area of tension: political guidelines
Starting point is the question: Do students answer physics tasks differently depending on their gender identity, migration history and disability? Students in seventh and eighth grade might answer energy tasks in exactly the same manner, so we might not find any differences. In this case, it would be over-regulation to politically prescribe how training data must be composed in relation to gender identity and thus an unnecessary bureaucratic hurdle. Alternatively, is there a difference in the answers? If so, regulation may be necessary to avoid discriminating against students with AI. Our initial unpublished results suggest that bias exists in AI for physics education if not actively addressed. Through our research, we can identify where this bias can be counteracted particularly effectively and efficiently.
How bias gets into AI
There are several phases in the life cycle of AI systems in which bias can arise. We focus on the phases of data preparation and model training, as they are the most likely to have a regulatory impact on physics education and have promising potential.
Representation bias
Bias can occur in the data preparation and model training phase if the data used is not representative of the subsequent application. This is known as representation bias. An example of this is when an AI system is only trained with data from grammar schools, but is later to be used in other types of schools such as comprehensive schools. The AI could then perform worse at these other school types because the differences between the schools were not taken into account in the training data.
Coding bias
Coding bias describes how students' answers are evaluated by people and that some students are systematically rated worse than they are. An example: Answers from female students are systematically rated lower than the answers from male students by a coding person, which can happen to all of us unintentionally due to the influence of social prejudices. If an AI is then trained with this evaluation, this AI has a bias and also systematically rates the answers of female students lower.
Evaluation bias
In addition, we look at the evaluation bias, where the bias arises because a test method is designed so that existing discrimination is not uncovered. If an AI is only tested to see whether a class learns more on average than without AI, for example, this says nothing about the bias. Male students in this class might learn much more on average with AI than female students. A separate evaluation of the groups of students based on gender identity would be required in addition to an overall evaluation.
Strategies for minimizing bias: Our research methods
Currently, we are examining whether it makes a difference which data is used to train an AI. To do this, we train the AI with data from female students only, then with 10% data from male students and 90% data from female students, then with 20% to 80%, until we finally train it with 100% data from male students. Finally, we evaluate whether - depending on the training data - the AI performs worse for female students than for male students. Our initial results indicate that the composition of the training data has an effect, but is not solely responsible for bias in AI.
In addition, we look at the question of how a coding bias can be addressed. For this purpose, we have the same answers from students evaluated by several coders. We then look at whether the AI - depending on who coded the data - works differently for male and female students. If it does make a difference, there appears to be a coding bias. This coding bias must then be counteracted before the AI is actually trained. We are still in the process of evaluating the first data sets.
Lastly, we look at the question of how to address representation bias. First, we try to use the students' answers to predict their gender identity. When this works, there are obviously gender-based patterns in the students' answers. The better this works, the stronger the patterns will be. An AI learns patterns from training data. So, without taking other aspects into account, the following initially applies: The greater the gender-based patterns in the students' answers, the greater the risk that the AI will use these patterns as a shortcut. This would be problematic because it represents discrimination and not an evaluation based on quality criteria. Such a risk assessment can be used to set higher or lower requirements for the evaluation of AI. Our initial results indicate that a risk assessment works. In other words, the better an AI can predict the gender identity of students, the greater its gender-based bias.
Conclusion: Equitable AI requires political regulation
The use of AI in physics lessons is increasing which is good as it supports learning. However, we are also working to ensure that learning is supported equally for all students. The aim is to ensure that the already existing underrepresentation of female students, for example, is not exacerbated. AI in physics lessons is not automatically without bias, on the contrary: it requires proactive work to develop AI that has no bias
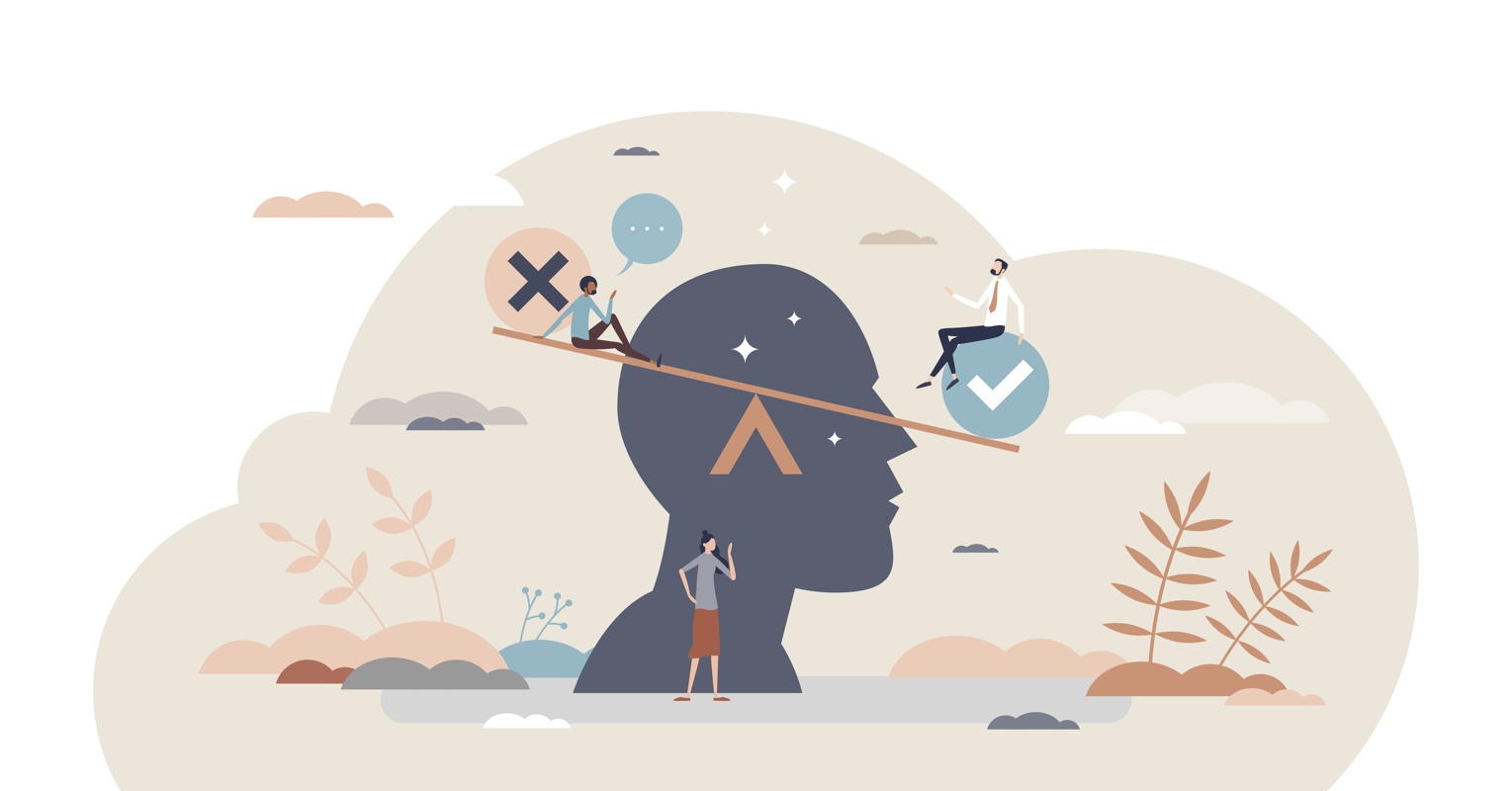
About the authors:
Adrian Grimm is a research scientist in the Department of Physics Education at the IPN. In his research, he focuses in particular on the question of how science lessons can be designed to be inviting for all students, so that historically grown inequalities are actively reduced along diversity dimensions. grimm@leibniz-ipn.de
Mastodon: @AdrianGrimm@digitalcourage.social
Dr. Anneke Steegh is a postdoctoral research scientist at the IPN in the Department of Chemistry Education. She conducts research on STEM identity and marginalized identities in STEM education. steegh@leibniz-ipn.de
Further literature:
Grimm, A., Steegh, A., Çolakoğlu, J., Kubsch, M., & Neumann, K. (2023). Positioning responsible learning analytics in the context of STEM identities of under-served students. Frontiers in Education, 7. https://doi.org/10.3389/feduc.2022.1082748
Grimm, A., Steegh, A., Kubsch, M., & Neumann, K. (2023). Learning Analytics in Physics Education: Equity- Focused Decision-Making Lacks Guidance! Journal of Learning Analytics, 10(1), 71–84. https://doi.org/10.18608/jla.2023.7793
Mühling, A. (2024). Die Lernumgebungen des KI-Labors. IPN Journal, 2024(11), 18–20.